Discovering 3D Parts from Image Collections
Chun-Han Yao Wei-Chih Hung Varun Jampani Ming-Hsuan Yang
Abstract
Reasoning 3D shapes from 2D images is an essential yet challenging task, especially when only single-view images are at our disposal. While an object can have a complicated shape, individual parts are usually close to geometric primitives and thus are easier to model. Furthermore, parts provide a mid-level representation that is robust to appearance variations across objects in a particular category. In this work, we tackle the problem of 3D part discovery from only 2D image collections. Instead of relying on manually annotated parts for supervision, we propose a self-supervised approach, latent part discovery (LPD). Our key insight is to learn a novel part shape prior that allows each part to fit an object shape faithfully while constrained to have simple geometry. Extensive experiments on the synthetic ShapeNet, PartNet, and real-world Pascal 3D+ datasets show that our method discovers consistent object parts and achieves favorable reconstruction accuracy compared to the existing methods with the same level of supervision.
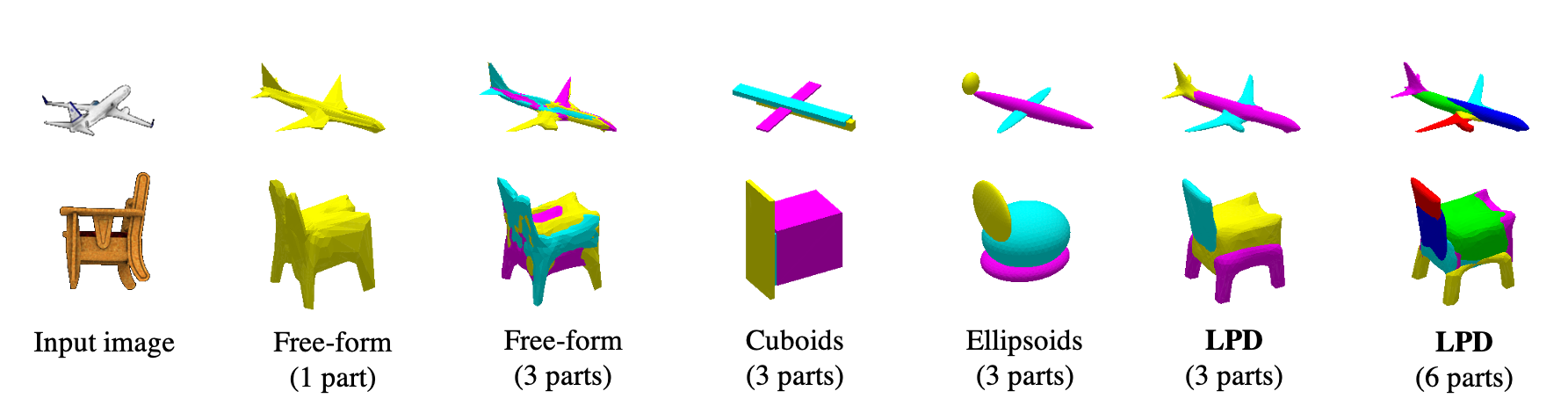
Our method (LPD) enables self-supervised 3D part discovery while learning to reconstruct object shapes from single-view images. Compared to other methods using different part constraints, LPD discovers more faithful and consistent parts, which improve the reconstruction quality and allow part reasoning/manipulation.
Paper & Code
Video
Bibtex
@inproceedings{yao2021discovering,
title={Discovering 3D Parts from Image Collections},
author={Yao, Chun-Han and Hung, Wei-Chih and Jampani, Varun and Yang, Ming-Hsuan},
booktitle={Proceedings of the IEEE/CVF International Conference on Computer Vision},
year={2021}
}